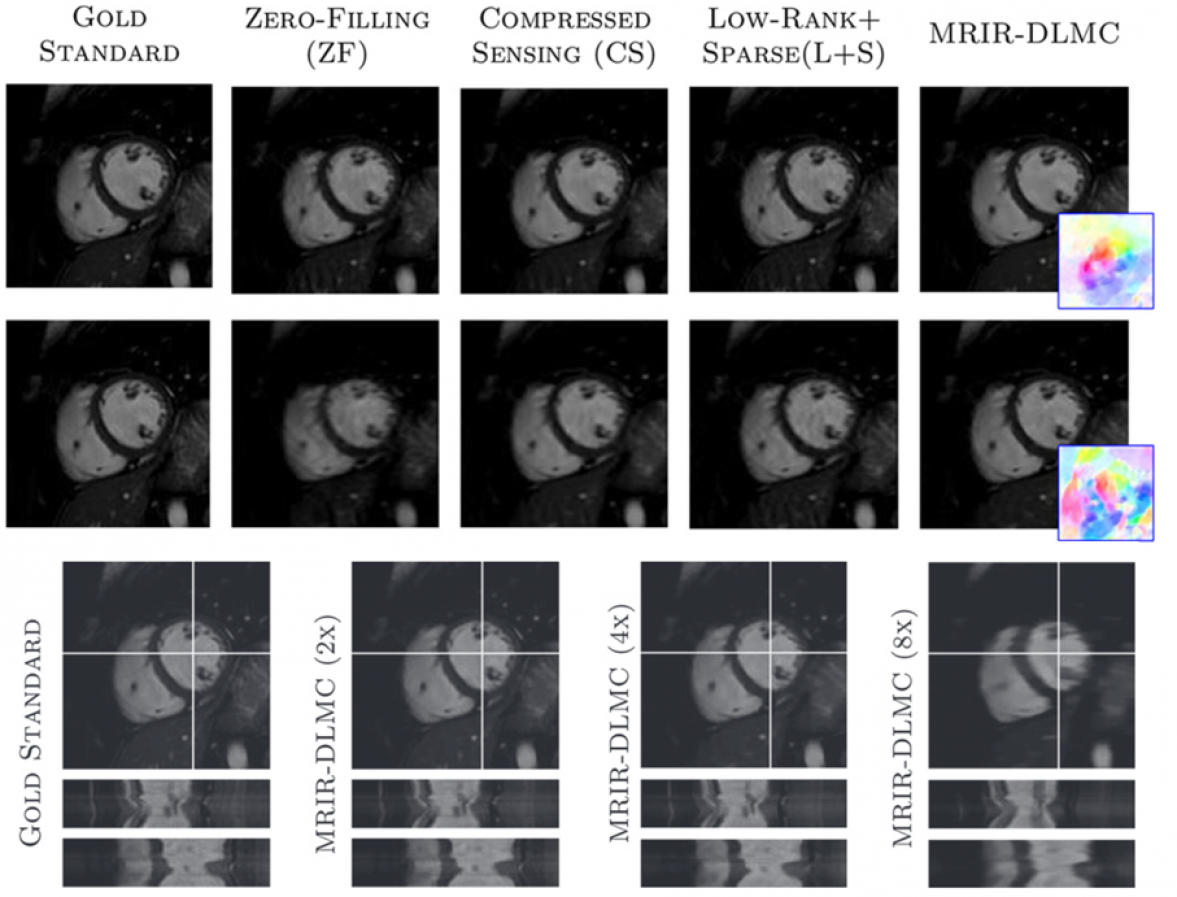
Researcher: Angelica Aviles Rivero, Veronica Corona, Noémie Debroux, Carola-Bibiane Schönlieb
However, in most recent years, there has been a great interest for improving medical image reconstruction by using what is called multi-tasking models (also known as joint models). The central idea of this perspective is that by sharing representation between tasks and carefully intertwining them, one can create synergies across challenging problems and reduce error propagation, which results in boosting the accuracy of the outcomes whilst achieving better generalisation capabilities. In this project, we aim to develop computationally tractable and mathematically well-motivated multi-task frameworks for improving image reconstruction. To do this, we investigate how reconstruction can be improved when other close related tasks are used– for example, image registration (motion estimation) and super-resolution.