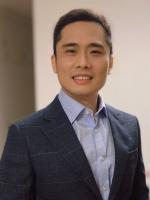
Dr Chao Li is a Principal Research Fellow with expertise in both healthcare and AI innovation, with comprehensive experience in developing image-based AI and multi-omics approaches to model neurological diseases. Dr Li is particularly interested in developing cost-effective AI models and translating these models into healthcare management to promote personalised medicine. His research is surrounding the below themes: 1. Image-based AI for precision mental health. 2. Image-based AI for precision surgical and interventional oncology. 3. Multi-omics AI for disease characterisation and precision medicine. 4. Efficacy and safety assessment of AI innovations for clinical translation and enterprise.
Publications
Multi-Modal Learning for Predicting the Genotype of Glioma
– IEEE Trans Med Imaging
(2023)
42,
1
(doi: 10.1109/tmi.2023.3244038)
G-CNN: Adaptive Geometric Convolutional Neural Networks for MRI-Based Skull Stripping
– Lecture Notes in Computer Science (including subseries Lecture Notes in Artificial Intelligence and Lecture Notes in Bioinformatics)
(2023)
14243,
21
(doi: 10.1007/978-3-031-45087-7_3)
DisC-Diff: Disentangled Conditional Diffusion Model for Multi-contrast MRI Super-Resolution
– Lecture Notes in Computer Science (including subseries Lecture Notes in Artificial Intelligence and Lecture Notes in Bioinformatics)
(2023)
14229,
387
(doi: 10.1007/978-3-031-43999-5_37)
Multi-task Learning of Histology and Molecular Markers for Classifying Diffuse Glioma
– Lecture Notes in Computer Science (including subseries Lecture Notes in Artificial Intelligence and Lecture Notes in Bioinformatics)
(2023)
14226 LNCS,
551
(doi: 10.1007/978-3-031-43990-2_52)
CoLa-Diff: Conditional Latent Diffusion Model for Multi-modal MRI Synthesis
– Lecture Notes in Computer Science (including subseries Lecture Notes in Artificial Intelligence and Lecture Notes in Bioinformatics)
(2023)
14229,
398
(doi: 10.1007/978-3-031-43999-5_38)
Multi-task Learning of Histology and Molecular Markers for Classifying
Diffuse Glioma
(2023)
DisC-Diff: Disentangled Conditional Diffusion Model for Multi-Contrast
MRI Super-Resolution
(2023)
CoLa-Diff: Conditional Latent Diffusion Model for Multi-Modal MRI
Synthesis
(2023)
Expectation-Maximization Regularised Deep Learning for Tumour Segmentation
– 2023 IEEE 20th International Symposium on Biomedical Imaging (ISBI)
(2023)
00,
1
Structural connectome quantifies tumour invasion and predicts survival in glioblastoma patients
– Brain
(2022)
146,
1714
(doi: 10.1093/brain/awac360)
- 1 of 5