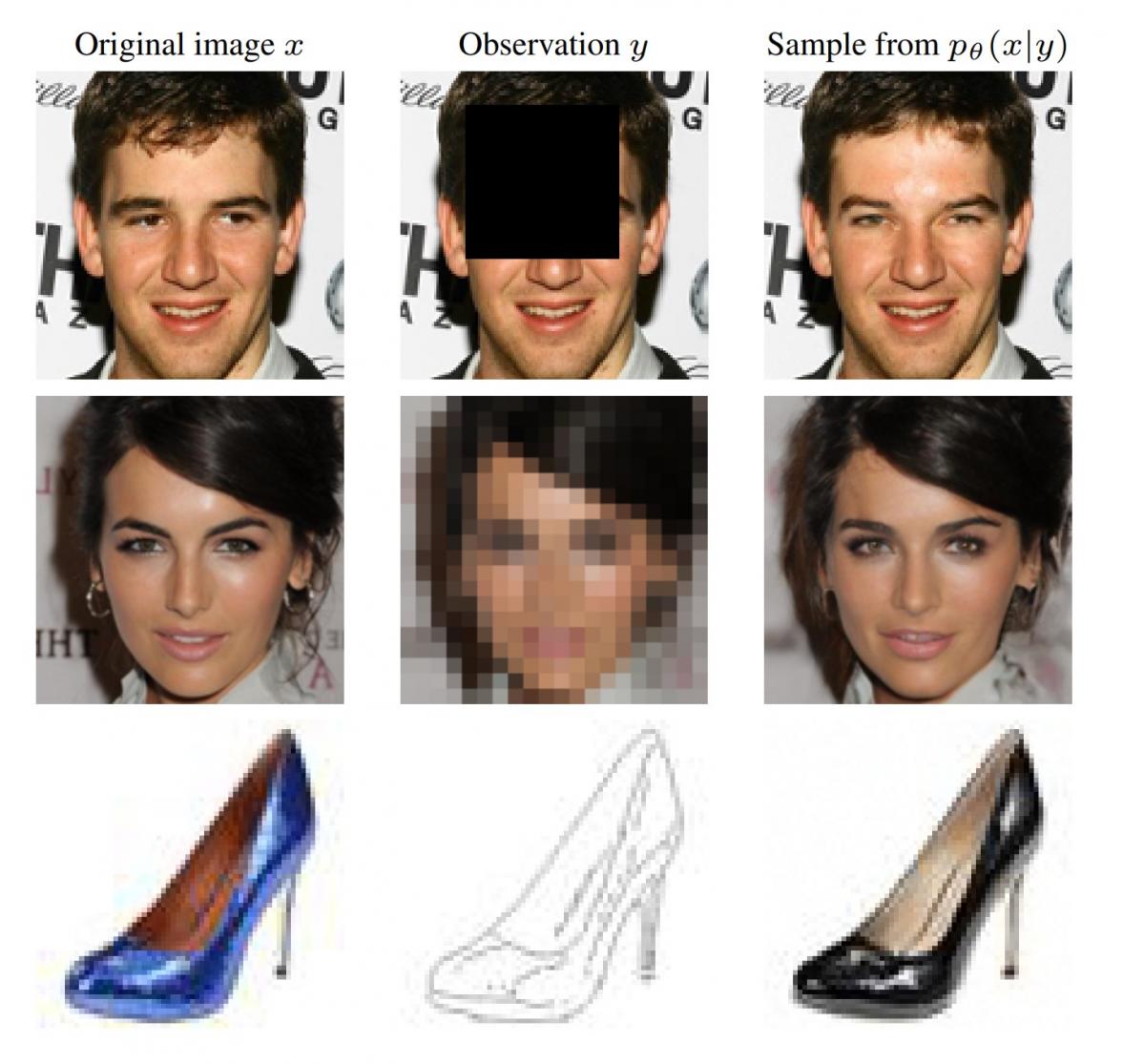
Researcher: Georgios Batzolis, Jan Stanczuk, Carola-Bibiane Schonlieb and Christian Etmann.
Deep generative modelling became one of the central areas of deep learning with many successful applications. In recent years much progress has been made in unconditional and conditional image generation. The most prominent approaches are auto-regressive models, variational autoencoders (VAEs), normalizing flows and generative adversarial networks (GANs). Despite their success, each of the above methods suffers from important limitations. Recently, continuous-time approach based on stochastic differential equations called score-based diffusion models achieved state-of-the-art performance in likelihood estimation and unconditional image generation, surpassing even the celebrated success of GANs. In this work, we examine how score-based diffusion models can be applied to conditional image generation. We conduct a review and classification of existing approaches and perform a systematic comparison to find the best way of estimating the conditional score. We provide a proof of validity for the conditional denoising estimator (which has been used without justification), and we thereby provide a firm theoretical foundation for using it in future research. Moreover, we extend the original framework to support multi-speed diffusion, where different parts of the input tensor diffuse according to different speeds. This allows us to introduce a novel estimator of the conditional score and opens an avenue for further research.
Related Papers: Conditional Image Generation with Score-Based Diffusion Models, arXiv preprint arXiv:2111.13606 (2021).