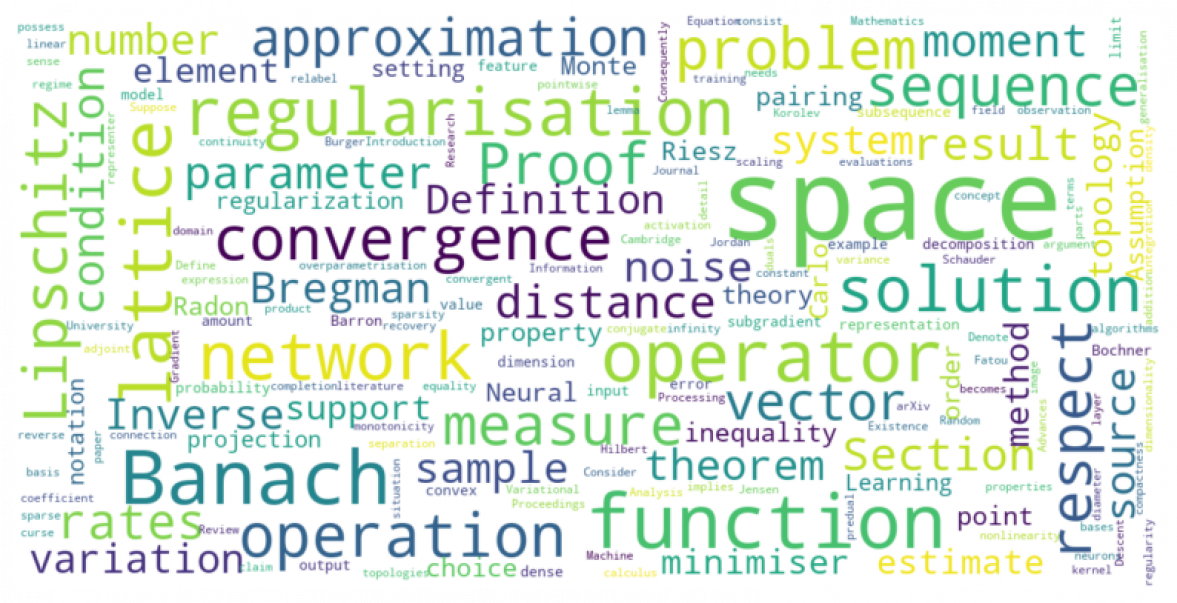
Researcher: Yury Korolev, and Carola-Bibiane Schönlieb
Universal approximation properties of various types of neural networks have been known since the late 1980’s. However, it has also been shown that the approximation rates in terms of the number of neurons scale exponentially with the dimension of the input space. However, certain types of functions can be approximated with dimension-independent Monte-Carlo rates. The functional-analytic study of the spaces of such functions has recently become an active area of research.
When neural networks are used in inherently infinite-dimensional applications such as inverse problems and imaging, they need to be considered as nonlinear operators between infinite-dimensional spaces, rather than functions between Euclidean spaces (even if these are high-dimensional). The generalisation from high but finite dimension to infinite dimension is far from being trivial and requires advanced functional-analytic techniques.
The goal of this project is to advance the understanding of neural networks in the infinite-dimensional setting and to use this understanding to construct more stable and efficient numerical algorithms.