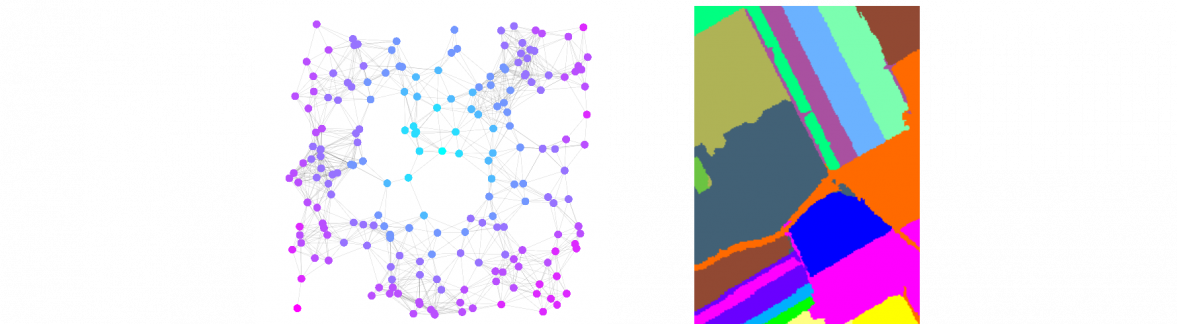
Researcher: Angelica Aviles Rivero, Philip Sellars, David Coomes, Nicolas Papadakis, Carola-Bibiane Schönlieb
In Hyperspectral Image Classification there is a major problem, collecting labelled data samples takes a lot of time and costs a lot of money. Therefore, supervised learning methods are often inappropriate to use as they require a large quality labelled data set to avoid over-fitting. To counter this, we are investigating the application of semi-supervised learning methods to hyperspectral classification. Semi-supervised learning methods additionally make use of un-labelled data for training and as such can performance better than using only the labelled data. We hope that by applying semi-supervised learning methods we can reduce the need for large amounts of training data.
Watch below the video produced for this project as part of the CCIMI video contest.