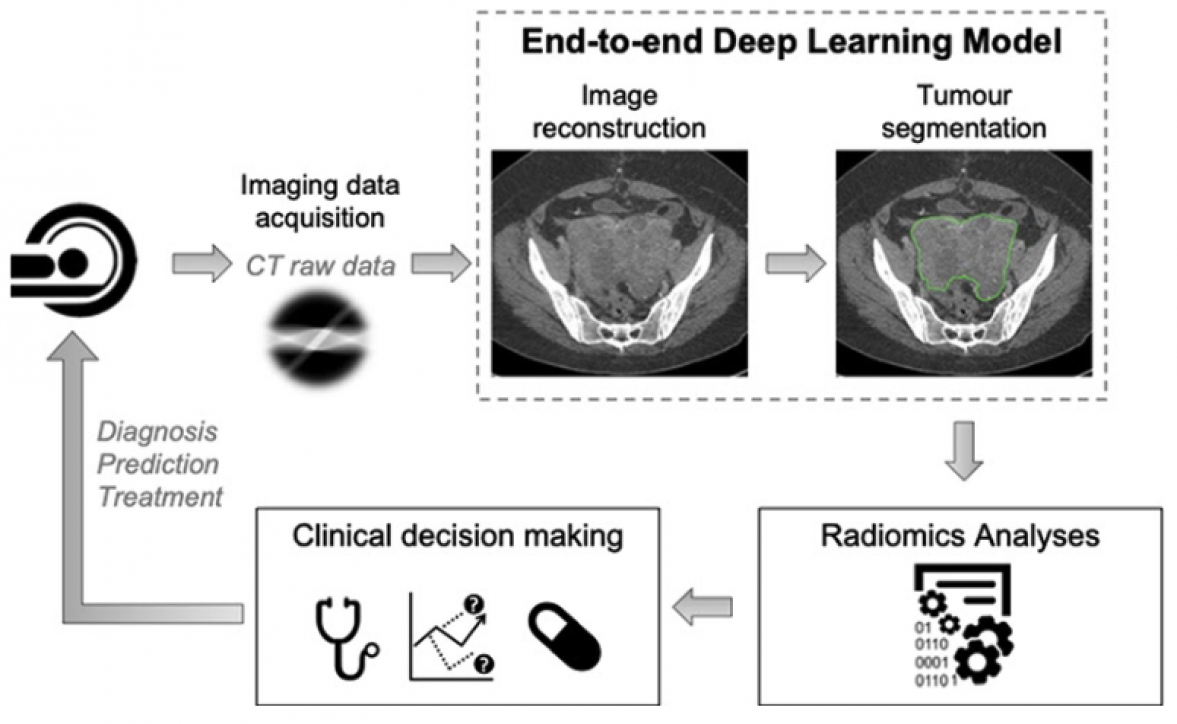
Researchers: Thomas Buddenkotte, Subhadip Mukherjee, Carola-Bibiane Schönlieb and Evis Sala
Approximately 7,400 women are diagnosed with ovarian cancer every year in the UK and 300,000 worldwide. Medical imaging is routinely used for diagnosis and treatment monitoring of patients with solid tumours and is currently the only available technique for noninvasively assessing treatment response taking into account the overall tumour burden and its spatial and temporal heterogeneity, which in turn are associated with outcome. Currently, image segmentation and evaluation are mostly performed manually and semiquantitatively. The advent of modern computing and the availability of large digital image databanks have led to development of quantitative, data-driven, machine-learning-based medical image analysis methods (radiomics) which have shown great promise in facilitating a deeper understanding of tumour biology, capturing tumour heterogeneity and monitoring tumour evolution and therapy response. However, the robustness and generalisability of the radiomics results depend on standardisation of CT instrumentation, data acquisition protocols, choice of reconstruction algorithm, pixel size, etc, which typically vary widely in routine clinical practice.
This project addresses the above challenges and provides a robust image analysis pipeline that improves significantly on the performance and predictive power of current radiomics metrics, ultimately improving clinical decisions and patient outcomes: 1. It exploits the power of novel deep neural networks to produce uniform and homogeneous images. 2. It seamlessly integrates image reconstruction with subsequent steps like semantic segmentation (pixel-wise classification) and radiomics analysis. 3. It is integrated in patient’s clinical workflow, providing accurate dynamic quantification of tumour burden that can be used to make real time treatment decisions in both the clinic and MDT settings.
This project has its own website.