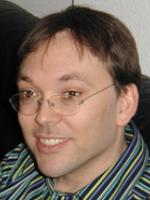
Career
- 1997-2000 Wellcome Trust Fellow in Mathematical Biology, Edinburgh
- 2000-2001 Lecturer, School of Informatics, Edinburgh
- 2001-2004 Wellcome Trust Travelling Fellowship, St Louis and Edinburgh
- 2004-2006 Lecturer, DAMTP
- 2006-2015 Senior Lecturer, DAMTP
- 2015- Reader. DAMTP
Research
Stephen Eglen is a computational neuroscientist: he uses computational methods to study the development of the nervous system, using mostly the retina and other parts of the visual pathway as a model system. He is particularly interested in questions of structural and functional development:
Structural development: how do retinal neurons acquire their positional information within a circuit?
Functional development: what are the mechanisms by which neurons make contact with each other, to perform functioning circuits?
Selected Publications
Please see my publications page
Publications
MEA-NAP compares microscale functional connectivity, topology, and network dynamics in organoid or monolayer neuronal cultures.
(2024)
(doi: 10.1101/2024.02.05.578738)
Bayesian model selection for multilevel models using integrated likelihoods
– PLoS One
(2023)
18,
e0280046
(doi: 10.48550/arxiv.2207.02144)
Bayesian model selection for multilevel models using integrated likelihoods
(2022)
(doi: 10.48550/arxiv.2207.02144)
Sepsis-3 criteria in AmsterdamUMCdb: open-source code implementation.
– GigaByte (Hong Kong, China)
(2022)
2022,
gigabyte45
(doi: 10.46471/gigabyte.45)
Analysis of Activity Dependent Development of Topographic Maps in Neural Field Theory with Short Time Scale Dependent Plasticity
– Mathematical Neuroscience and Applications
(2022)
2,
(doi: 10.46298/mna.8390)
Homophilic wiring principles underpin neuronal network topologyin vitro
(2022)
(doi: 10.1101/2022.03.09.483605)
Causality indices for bivariate time series data: a comparative review of performance
– Chaos: An Interdisciplinary Journal of Nonlinear Science
(2021)
31,
083111
(doi: 10.1063/5.0053519)
Causality indices for bivariate time series data: A comparative review of performance
– Chaos An Interdisciplinary Journal of Nonlinear Science
(2021)
31,
083111
(doi: 10.1063/5.0053519)
DeepClean: Self-Supervised Artefact Rejection for Intensive Care Waveform Data Using Deep Generative Learning
– Acta Neurochirurgica: Supplementum
(2021)
131,
235
(doi: 10.1007/978-3-030-59436-7_45)
- 1 of 12